Q1. What are the different types of Machine Learning?
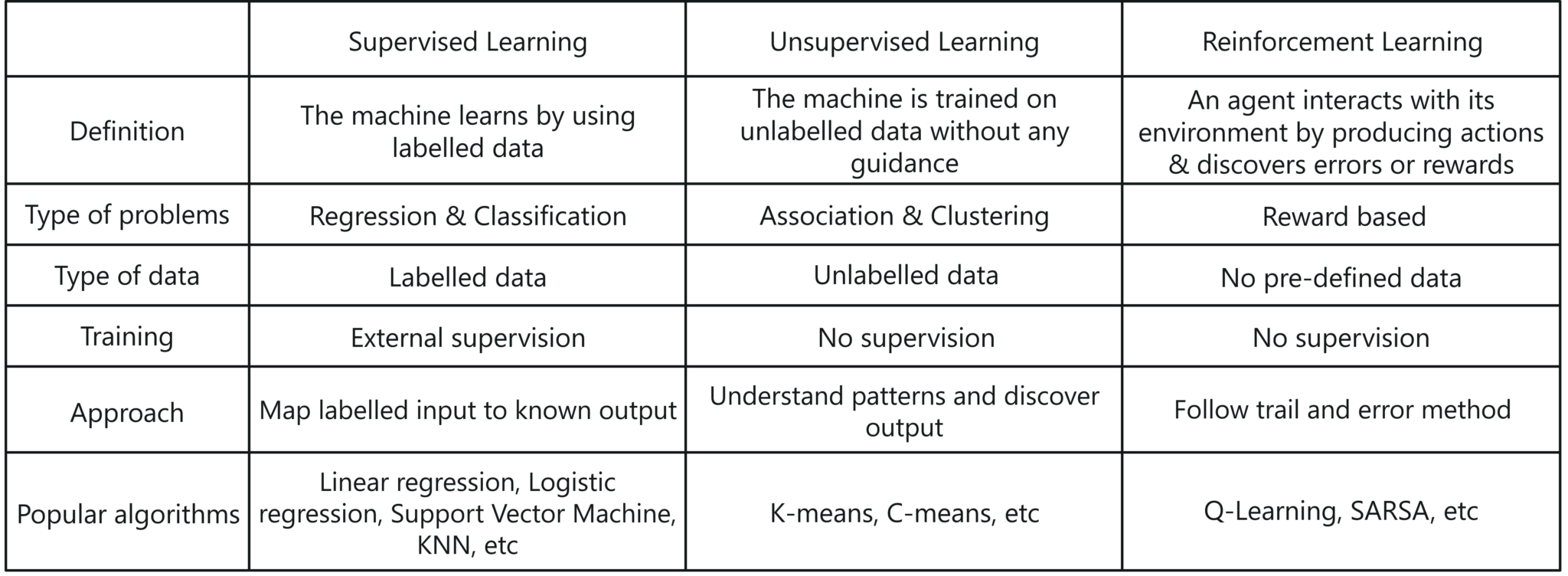
There are three ways in which machines learn:
- Supervised Learning
- Unsupervised Learning
- Reinforcement Learning
Supervised Learning:
Supervised learning is a method in which the machine learns using labeled data.
- It is like learning under the guidance of a teacher
- Training dataset is like a teacher which is used to train the machine
- Model is trained on a pre-defined dataset before it starts making decisions when given new data
Unsupervised Learning:
Unsupervised learning is a method in which the machine is trained on unlabelled data or without any guidance
- It is like learning without a teacher.
- Model learns through observation & finds structures in data.
- Model is given a dataset and is left to automatically find patterns and relationships in that dataset by creating clusters.
Reinforcement Learning:
Reinforcement learning involves an agent that interacts with its environment by producing actions & discovers errors or rewards.
- It is like being stuck in an isolated island, where you must explore the environment and learn how to live and adapt to the living conditions on your own.
- Model learns through the hit and trial method
- It learns on the basis of reward or penalty given for every action it performs
Q2. How would you explain Machine Learning to a school-going kid?
- Suppose your friend invites you to his party where you meet total strangers. Since you have no idea about them, you will mentally classify them on the basis of gender, age group, dressing, etc.
- In this scenario, the strangers represent unlabeled data and the process of classifying unlabeled data points is nothing but unsupervised learning.
- Since you didn’t use any prior knowledge about people and classified them on-the-go, this becomes an unsupervised learning problem.
Q3. How does Deep Learning differ from Machine Learning?
Deep Learning | Machine Learning |
![]() | ![]()
Machine Learning is all about algorithms that parse data, learn from that data, and then apply what they’ve learned to make informed decisions.
|
Q4. Explain Classification and Regression
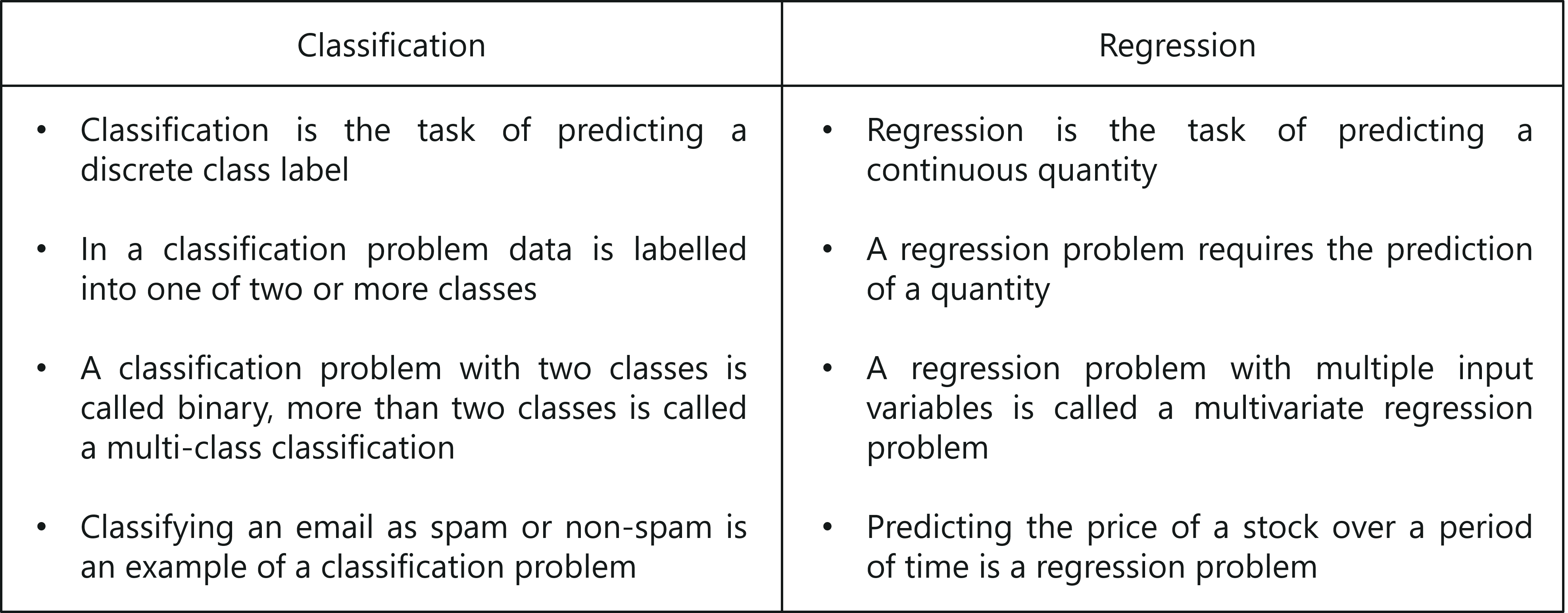
Q5. What do you understand by selection bias?
- It is a statistical error that causes a bias in the sampling portion of an experiment.
- The error causes one sampling group to be selected more often than other groups included in the experiment.
- Selection bias may produce an inaccurate conclusion if the selection bias is not identified.
Q6. What do you understand by Precision and Recall?
Let me explain you this with an analogy:
- Imagine that, your girlfriend gave you a birthday surprise every year for the last 10 years. One day, your girlfriend asks you: ‘Sweetie, do you remember all the birthday surprises from me?’
- To stay on good terms with your girlfriend, you need to recall all the 10 events from your memory. Therefore, recall is the ratio of the number of events you can correctly recall, to the total number of events.
- If you can recall all 10 events correctly, then, your recall ratio is 1.0 (100%) and if you can recall 7 events correctly, your recall ratio is 0.7 (70%)
However, you might be wrong in some answers.
- For example, let’s assume that you took 15 guesses out of which 10 were correct and 5 were wrong. This means that you can recall all events but not so precisely
- Therefore, precision is the ratio of a number of events you can correctly recall, to the total number of events you can recall (mix of correct and wrong recalls).
- From the above example (10 real events, 15 answers: 10 correct, 5 wrong), you get 100% recall but your precision is only 66.67% (10 / 15)
Q7. Explain false negative, false positive, true negative and true positive with a simple example.
Let’s consider a scenario of a fire emergency:
- True Positive: If the alarm goes on in case of a fire.
Fire is positive and prediction made by the system is true. - False Positive: If the alarm goes on, and there is no fire.
System predicted fire to be positive which is a wrong prediction, hence the prediction is false. - False Negative: If the alarm does not ring but there was a fire.
System predicted fire to be negative which was false since there was fire. - True Negative: If the alarm does not ring and there was no fire.
The fire is negative and this prediction was true.
Q8. What is a Confusion Matrix?
A confusion matrix or an error matrix is a table which is used for summarizing the performance of a classification algorithm.
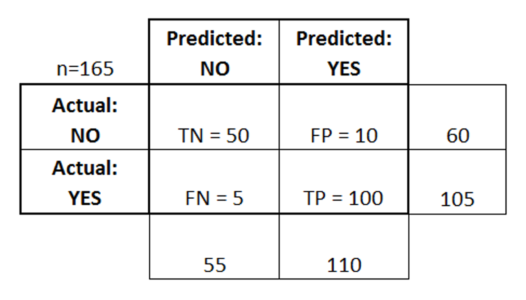
Consider the above table where:
- TN = True Negative
- TP = True Positive
- FN = False Negative
- FP = False Positive
Q9. What is the difference between inductive and deductive learning?
- Inductive learning is the process of using observations to draw conclusions
- Deductive learning is the process of using conclusions to form observations
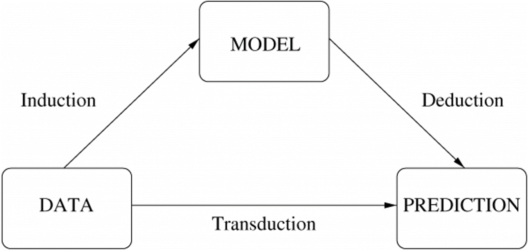
Q10. How is KNN different from K-means clustering?
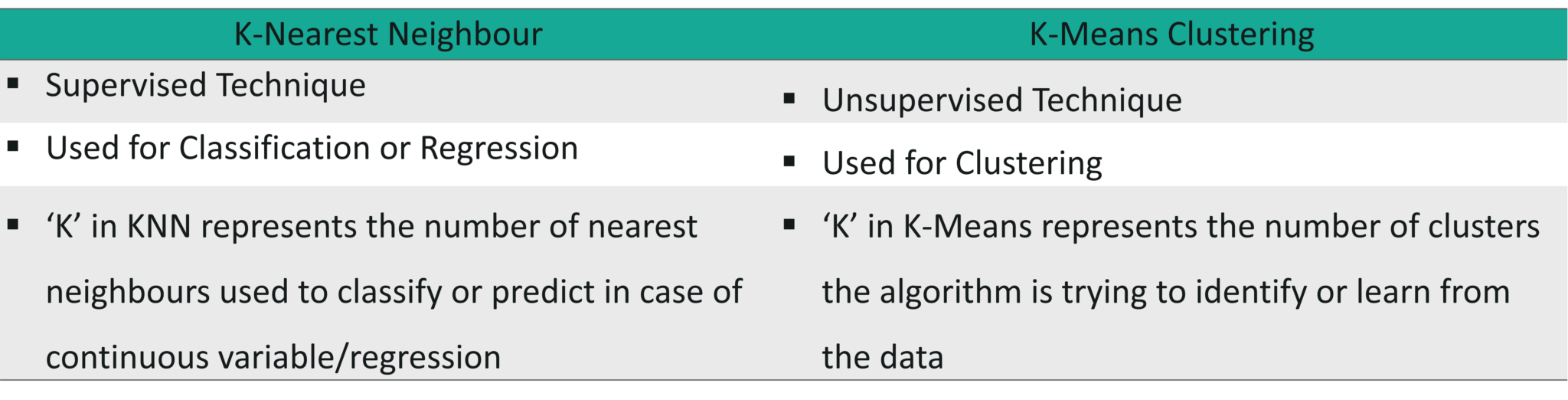
Q11. What is ROC curve and what does it represent?
Receiver Operating Characteristic curve (or ROC curve) is a fundamental tool for diagnostic test evaluation and is a plot of the true positive rate (Sensitivity) against the false positive rate (Specificity) for the different possible cut-off points of a diagnostic test.
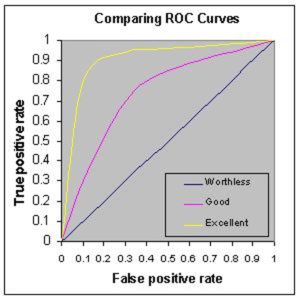
- It shows the tradeoff between sensitivity and specificity (any increase in sensitivity will be accompanied by a decrease in specificity).
- The closer the curve follows the left-hand border and then the top border of the ROC space, the more accurate the test.
- The closer the curve comes to the 45-degree diagonal of the ROC space, the less accurate the test.
- The slope of the tangent line at a cutpoint gives the likelihood ratio (LR) for that value of the test.
- The area under the curve is a measure of test accuracy.
Q12. What’s the difference between Type I and Type II error?
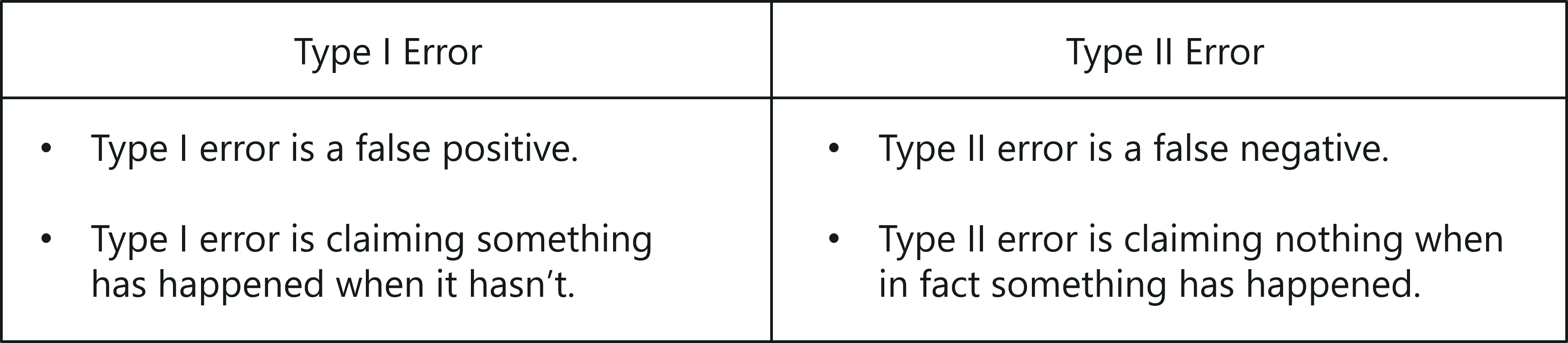
Q13. Is it better to have too many false positives or too many false negatives? Explain.
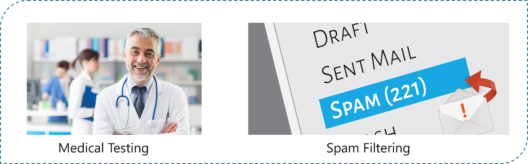
It depends on the question as well as on the domain for which we are trying to solve the problem. If you’re using Machine Learning in the domain of medical testing, then a false negative is very risky, since the report will not show any health problem when a person is actually unwell. Similarly, if Machine Learning is used in spam detection, then a false positive is very risky because the algorithm may classify an important email as spam.
Q14. Which is more important to you – model accuracy or model performance?
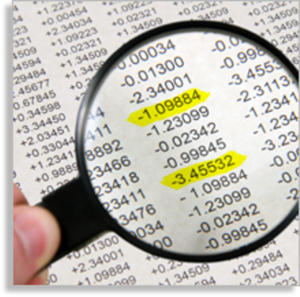
Well, you must know that model accuracy is only a subset of model performance. The accuracy of the model and performance of the model are directly proportional and hence better the performance of the model, more accurate are the predictions.
Q15. What is the difference between Gini Impurity and Entropy in a Decision Tree?
- Gini Impurity and Entropy are the metrics used for deciding how to split a Decision Tree.
- Gini measurement is the probability of a random sample being classified correctly if you randomly pick a label according to the distribution in the branch.
- Entropy is a measurement to calculate the lack of information. You calculate the Information Gain (difference in entropies) by making a split. This measure helps to reduce the uncertainty about the output label.
Q16. What is the difference between Entropy and Information Gain?
- Entropy is an indicator of how messy your data is. It decreases as you reach closer to the leaf node.
- The Information Gain is based on the decrease in entropy after a dataset is split on an attribute. It keeps on increasing as you reach closer to the leaf node.
Q17. What is Overfitting? And how do you ensure you’re not overfitting with a model?
Over-fitting occurs when a model studies the training data to such an extent that it negatively influences the performance of the model on new data.
This means that the disturbance in the training data is recorded and learned as concepts by the model. But the problem here is that these concepts do not apply to the testing data and negatively impact the model’s ability to classify the new data, hence reducing the accuracy on the testing data.
Three main methods to avoid overfitting:
- Collect more data so that the model can be trained with varied samples.
- Use ensembling methods, such as Random Forest. It is based on the idea of bagging, which is used to reduce the variation in the predictions by combining the result of multiple Decision trees on different samples of the data set.
- Choose the right algorithm.
Q18.Explain Ensemble learning technique in Machine Learning.
Ensemble learning is a technique that is used to create multiple Machine Learning models, which are then combined to produce more accurate results. A general Machine Learning model is built by using the entire training data set. However, in Ensemble Learning the training data set is split into multiple subsets, wherein each subset is used to build a separate model. After the models are trained, they are then combined to predict an outcome in such a way that the variance in the output is reduced.
Q19. What is bagging and boosting in Machine Learning?
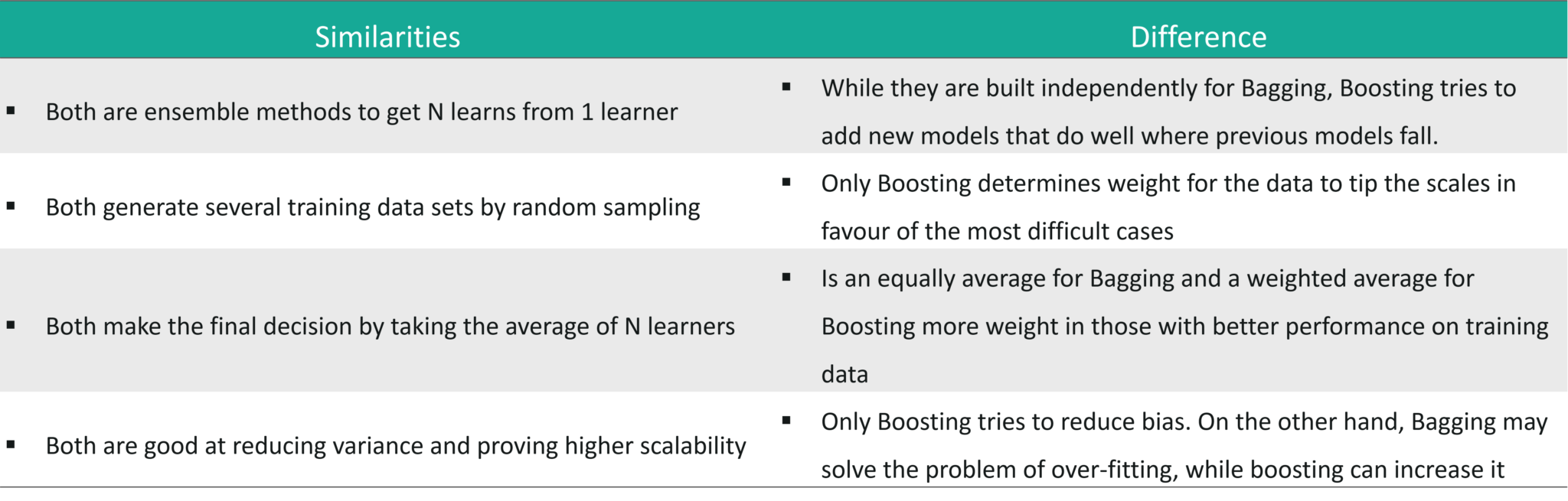
Q20. How would you screen for outliers and what should you do if you find one?
The following methods can be used to screen outliers:
- Boxplot: A box plot represents the distribution of the data and its variability. The box plot contains the upper and lower quartiles, so the box basically spans the Inter-Quartile Range (IQR). One of the main reasons why box plots are used is to detect outliers in the data. Since the box plot spans the IQR, it detects the data points that lie outside this range. These data points are nothing but outliers.
- Probabilistic and statistical models: Statistical models such as normal distribution and exponential distribution can be used to detect any variations in the distribution of data points. If any data point is found outside the distribution range, it is rendered as an outlier.
- Linear models: Linear models such as logistic regression can be trained to flag outliers. In this manner, the model picks up the next outlier it sees.
- Proximity-based models: An example of this kind of model is the K-means clustering model wherein, data points form multiple or ‘k’ number of clusters based on features such as similarity or distance. Since similar data points form clusters, the outliers also form their own cluster. In this way, proximity-based models can easily help detect outliers.
How do you handle these outliers?
- If your data set is huge and rich then you can risk dropping the outliers.
- However, if your data set is small then you can cap the outliers, by setting a threshold percentile. For example, the data points that are above the 95th percentile can be used to cap the outliers.
- Lastly, based on the data exploration stage, you can narrow down some rules and impute the outliers based on those business rules.
Q21. What are collinearity and multicollinearity?
- Collinearity occurs when two predictor variables (e.g., x1 and x2) in a multiple regression have some correlation.
- Multicollinearity occurs when more than two predictor variables (e.g., x1, x2, and x3) are inter-correlated.
Q22. What do you understand by Eigenvectors and Eigenvalues?
- Eigenvectors: Eigenvectors are those vectors whose direction remains unchanged even when a linear transformation is performed on them.
- Eigenvalues: Eigenvalue is the scalar that is used for the transformation of an Eigenvector.
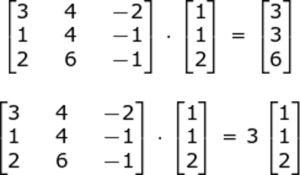
In the above example, 3 is an Eigenvalue, with the original vector in the multiplication problem being an eigenvector.Next
The Eigenvector of a square matrix A is a nonzero vector x such that for some number λ, we have the following:
Ax = λx,
where λ is an Eigenvalue
So, in our example, λ = 3 and X = [1 1 2]
So, in our example, λ = 3 and X = [1 1 2]
Q23. What is A/B Testing?
- A/B is Statistical hypothesis testing for randomized experiment with two variables A and B. It is used to compare two models that use different predictor variables in order to check which variable fits best for a given sample of data.
- Consider a scenario where you’ve created two models (using different predictor variables) that can be used to recommend products for an e-commerce platform.
- A/B Testing can be used to compare these two models to check which one best recommends products to a customer.
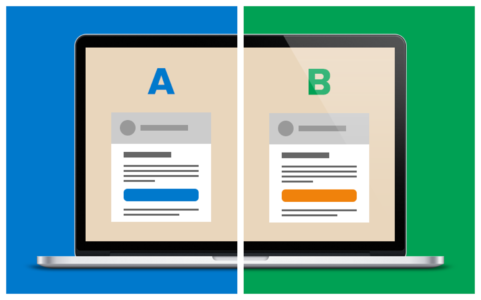
Q24. What is Cluster Sampling?
- It is a process of randomly selecting intact groups within a defined population, sharing similar characteristics.
- Cluster Sample is a probability sample where each sampling unit is a collection or cluster of elements.
- For example, if you’re clustering the total number of managers in a set of companies, in that case, managers (samples) will represent elements and companies will represent clusters.
Q25. Running a binary classification tree algorithm is quite easy. But do you know how the tree decides on which variable to split at the root node and its succeeding child nodes?
- Measures such as, Gini Index and Entropy can be used to decide which variable is best fitted for splitting the Decision Tree at the root node.
- We can calculate Gini as following:
Calculate Gini for sub-nodes, using the formula – sum of square of probability for success and failure (p^2+q^2). - Calculate Gini for split using weighted Gini score of each node of that split
- Entropy is the measure of impurity or randomness in the data, (for binary class):

- Entropy is zero when a node is homogeneous and is maximum when both the classes are present in a node at 50% – 50%. To sum it up, the entropy must be as low as possible in order to decide whether or not a variable is suitable as the root node.
Machine Learning With Python Questions
This set of Machine Learning interview questions deal with Python related Machine Learning questions.
Q1. Name a few libraries in Python used for Data Analysis and Scientific Computations.
Here is a list of Python libraries mainly used for Data Analysis:
- NumPy
- SciPy
- Pandas
- SciKit
- Matplotlib
- Seaborn
- Bokeh
Q2. Which library would you prefer for plotting in Python language: Seaborn or Matplotlib or Bokeh?
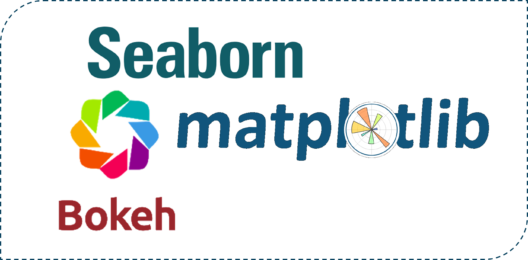
Python Libraries
It depends on the visualization you’re trying to achieve. Each of these libraries is used for a specific purpose:
- Matplotlib: Used for basic plotting like bars, pies, lines, scatter plots, etc
- Seaborn: Is built on top of Matplotlib and Pandas to ease data plotting. It is used for statistical visualizations like creating heatmaps or showing the distribution of your data
- Bokeh: Used for interactive visualization. In case your data is too complex and you haven’t found any “message” in the data, then use Bokeh to create interactive visualizations that will allow your viewers to explore the data themselves
Q3. How are NumPy and SciPy related?
- NumPy is part of SciPy.
- NumPy defines arrays along with some basic numerical functions like indexing, sorting, reshaping, etc.
- SciPy implements computations such as numerical integration, optimization and machine learning using NumPy’s functionality.
Q4. What is the main difference between a Pandas series and a single-column DataFrame in Python?
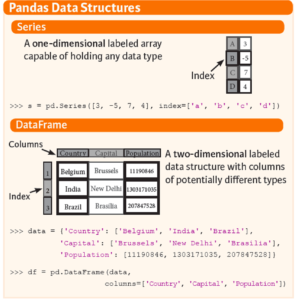
Pandas Series vs DataFrame
Q5. How can you handle duplicate values in a dataset for a variable in Python?
Consider the following Python code:
1
2
3
4
5
6
7
| bill_data=pd.read_csv("datasetsTelecom Data AnalysisBill.csv") bill_data.shape #Identify duplicates records in the data Dupes = bill_data.duplicated() sum(dupes) #Removing Duplicates bill_data_uniq = bill_data.drop_duplicates() |
Q6. Write a basic Machine Learning program to check the accuracy of a model, by importing any dataset using any classifier?
1
2
3
4
5
6
7
8
9
10
11
12
13
14
15
16
17
18
| #importing dataset import sklearn from sklearn import datasets iris = datasets.load_iris() X = iris.data Y = iris.target #splitting the dataset from sklearn.cross_validation import train_test_split X_train, Y_train, X_test, Y_test = train_test_split(X,Y, test_size = 0.5) #Selecting Classifier my_classifier = tree.DecisionTreeClassifier() My_classifier.fit(X_train, Y_train) predictions = my_classifier(X_test) #check accuracy From sklear.metrics import accuracy_score print accuracy_score(y_test, predictions) |
Machine Learning Scenario Based Questions
This set of Machine Learning interview questions deal with scenario-based Machine Learning questions.
Q1. You are given a data set consisting of variables having more than 30% missing values? Let’s say, out of 50 variables, 8 variables have missing values higher than 30%. How will you deal with them?
- Assign a unique category to the missing values, who knows the missing values might uncover some trend.
- We can remove them blatantly.
- Or, we can sensibly check their distribution with the target variable, and if found any pattern we’ll keep those missing values and assign them a new category while removing others.
Q2. Write an SQL query that makes recommendations using the pages that your friends liked. Assume you have two tables: a two-column table of users and their friends, and a two-column table of users and the pages they liked. It should not recommend pages you already like.
1
2
3
4
5
| SELECT f.user_id, l.page_id FROM friend f JOIN like l ON f.friend_id = l.user_id WHERE l.page_id NOT IN (SELECT page_id FROM like WHERE user_id = f.user_id) |
Q3. There’s a game where you are asked to roll two fair six-sided dice. If the sum of the values on the dice equals seven, then you win $21. However, you must pay $5 to play each time you roll both dice. Do you play this game? And in the follow-up: If he plays 6 times what is the probability of making money from this game?
- The first condition states that if the sum of the values on the 2 dices is equal to 7, then you win $21. But for all the other cases you must pay $5.
- First, let’s calculate the number of possible cases. Since we have two 6-sided dices, the total number of cases => 6*6 = 36.
- Out of 36 cases, we must calculate the number of cases that produces a sum of 7 (in such a way that the sum of the values on the 2 dices is equal to 7)
- Possible combinations that produce a sum of 7 is, (1,6), (2,5), (3,4), (4,3), (5,2) and (6,1). All these 6 combinations generate a sum of 7.
- This means that out of 36 chances, only 6 will produce a sum of 7. On taking the ratio, we get: 6/36 = 1/6
- So this suggests that we have a chance of winning $21, once in 6 games.
- So to answer the question if a person plays 6 times, he will win one game of $21, whereas for the other 5 games he will have to pay $5 each, which is $25 for all five games. Therefore, he will face a loss because he wins $21 but ends up paying $25.
Q4. We have two options for serving ads within Newsfeed:
1 – out of every 25 stories, one will be an ad
2 – every story has a 4% chance of being an ad
For each option, what is the expected number of ads shown in 100 news stories?
If we go with option 2, what is the chance a user will be shown only a single ad in 100 stories? What about no ads at all?
- The expected number of ads shown in 100 new stories for option 1 is equal to 4 (100/25 = 4).
- Similarly, for option 2, the expected number of ads shown in 100 new stories is also equal to 4 (4/100 = 1/25 which suggests that one out of every 25 stories will be an ad, therefore in 100 new stories there will be 4 ads)
- Therefore for each option, the total number of ads shown in 100 new stories is 4.
- The second part of the question can be solved by using Binomial distribution. Binomial distribution takes three parameters:
- The probability of success and failure, which in our case is 4%.
- The total number of cases, which is 100 in our case.
- The probability of the outcome, which is a chance that a user will be shown only a single ad in 100 stories
- p(single ad) = (0.96)^99*(0.04)^1
(note: here 0.96 denotes the chance of not seeing an ad in 100 stories, 99 denotes the possibility of seeing only 1 ad, 0.04 is the probability of seeing an ad once in 100 stories )
- In total, there are 100 positions for the ad. Therefore, 100 * p(single ad) = 7.03%
Q5. How would you predict who will renew their subscription next month? What data would you need to solve this? What analysis would you do? Would you build predictive models? If so, which algorithms?
- Let’s assume that we’re trying to predict renewal rate for Netflix subscription. So our problem statement is to predict which users will renew their subscription plan for the next month.
- Next, we must understand the data that is needed to solve this problem. In this case, we need to check the number of hours the channel is active for each household, the number of adults in the household, number of kids, which channels are streamed the most, how much time is spent on each channel, how much has the watch rate varied from last month, etc. Such data is needed to predict whether or not a person will continue the subscription for the upcoming month.
- After collecting this data, it is important that you find patterns and correlations. For example, we know that if a household has kids, then they are more likely to subscribe. Similarly, by studying the watch rate of the previous month, you can predict whether a person is still interested in a subscription. Such trends must be studied.
- The next step is analysis. For this kind of problem statement, you must use a classification algorithm that classifies customers into 2 groups:
- Customers who are likely to subscribe next month
- Customers who are not likely to subscribe next month
- Would you build predictive models? Yes, in order to achieve this you must build a predictive model that classifies the customers into 2 classes like mentioned above.
- Which algorithms to choose? You can choose classification algorithms such as Logistic Regression, Random Forest, Support Vector Machine, etc.
- Once you’ve opted the right algorithm, you must perform model evaluation to calculate the efficiency of the algorithm. This is followed by deployment.
Q6. How do you map nicknames (Pete, Andy, Nick, Rob, etc) to real names?
- This problem can be solved in n number of ways. Let’s assume that you’re given a data set containing 1000s of twitter interactions. You will begin by studying the relationship between two people by carefully analyzing the words used in the tweets.
- This kind of problem statement can be solved by implementing Text Mining using Natural Language Processing techniques, wherein each word in a sentence is broken down and co-relations between various words are found.
- NLP is actively used in understanding customer feedback, performing sentimental analysis on Twitter and Facebook. Thus, one of the ways to solve this problem is through Text Mining and Natural Language Processing techniques.
Q7. A jar has 1000 coins, of which 999 are fair and 1 is double headed. Pick a coin at random, and toss it 10 times. Given that you see 10 heads, what is the probability that the next toss of that coin is also a head?
- There are two ways of choosing a coin. One is to pick a fair coin and the other is to pick the one with two heads.
- Probability of selecting fair coin = 999/1000 = 0.999
Probability of selecting unfair coin = 1/1000 = 0.001 - Selecting 10 heads in a row = Selecting fair coin * Getting 10 heads + Selecting an unfair coin
- P (A) = 0.999 * (1/2)^10 = 0.999 * (1/1024) = 0.000976
P (B) = 0.001 * 1 = 0.001
P( A / A + B ) = 0.000976 / (0.000976 + 0.001) = 0.4939
P( B / A + B ) = 0.001 / 0.001976 = 0.5061 - Probability of selecting another head = P(A/A+B) * 0.5 + P(B/A+B) * 1 = 0.4939 * 0.5 + 0.5061 = 0.7531
Q8. Suppose you are given a data set which has missing values spread along 1 standard deviation from the median. What percentage of data would remain unaffected and Why?
Since the data is spread across the median, let’s assume it’s a normal distribution.
As you know, in a normal distribution, ~68% of the data lies in 1 standard deviation from mean (or mode, median), which leaves ~32% of the data unaffected. Therefore, ~32% of the data would remain unaffected by missing values.
As you know, in a normal distribution, ~68% of the data lies in 1 standard deviation from mean (or mode, median), which leaves ~32% of the data unaffected. Therefore, ~32% of the data would remain unaffected by missing values.
Q9. You are given a cancer detection data set. Let’s suppose when you build a classification model you achieved an accuracy of 96%. Why shouldn’t you be happy with your model performance? What can you do about it?
You can do the following:
- Add more data
- Treat missing outlier values
- Feature Engineering
- Feature Selection
- Multiple Algorithms
- Algorithm Tuning
- Ensemble Method
- Cross-Validation
Q10. You are working on a time series data set. Your manager has asked you to build a high accuracy model. You start with the decision tree algorithm since you know it works fairly well on all kinds of data. Later, you tried a time series regression model and got higher accuracy than the decision tree model. Can this happen? Why?
- Time series data is based on linearity while a decision tree algorithm is known to work best to detect non-linear interactions
- Decision tree fails to provide robust predictions. Why?
- The reason is that it couldn’t map the linear relationship as good as a regression model did.
- We also know that a linear regression model can provide a robust prediction only if the data set satisfies its linearity assumptions.
Q11. Suppose you found that your model is suffering from low bias and high variance. Which algorithm you think could tackle this situation and Why?
Type 1: How to tackle high variance?
- Low bias occurs when the model’s predicted values are near to actual values.
- In this case, we can use the bagging algorithm (eg: Random Forest) to tackle high variance problem.
- Bagging algorithm will divide the data set into its subsets with repeated randomized sampling.
- Once divided, these samples can be used to generate a set of models using a single learning algorithm. Later, the model predictions are combined using voting (classification) or averaging (regression).
Type 2: How to tackle high variance?
- Lower the model complexity by using regularization technique, where higher model coefficients get penalized.
- You can also use top n features from variable importance chart. It might be possible that with all the variable in the data set, the algorithm is facing difficulty in finding the meaningful signal.
Q12. You are given a data set. The data set contains many variables, some of which are highly correlated and you know about it. Your manager has asked you to run PCA. Would you remove correlated variables first? Why?
Possibly, you might get tempted to say no, but that would be incorrect.
Discarding correlated variables will have a substantial effect on PCA because, in the presence of correlated variables, the variance explained by a particular component gets inflated.
Discarding correlated variables will have a substantial effect on PCA because, in the presence of correlated variables, the variance explained by a particular component gets inflated.
Q13. You are asked to build a multiple regression model but your model R² isn’t as good as you wanted. For improvement, you remove the intercept term now your model R² becomes 0.8 from 0.3. Is it possible? How?
Yes, it is possible.
- The intercept term refers to model prediction without any independent variable or in other words, mean prediction
R² = 1 – ∑(Y – Y´)²/∑(Y – Ymean)² where Y´ is the predicted value. - In the presence of the intercept term, R² value will evaluate your model with respect to the mean model.
- In the absence of the intercept term (Ymean), the model can make no such evaluation,
- With large denominator,
Value of ∑(Y – Y´)²/∑(Y)² equation becomes smaller than actual, thereby resulting in a higher value of R².
Q14. You’re asked to build a random forest model with 10000 trees. During its training, you got training error as 0.00. But, on testing the validation error was 34.23. What is going on? Haven’t you trained your model perfectly?
- The model is overfitting the data.
- Training error of 0.00 means that the classifier has mimicked the training data patterns to an extent.
- But when this classifier runs on the unseen sample, it was not able to find those patterns and returned the predictions with more number of errors.
- In Random Forest, it usually happens when we use a larger number of trees than necessary. Hence, to avoid such situations, we should tune the number of trees using cross-validation.
Q15. ‘People who bought this also bought…’ recommendations seen on Amazon is based on which algorithm?
E-commerce websites like Amazon make use of Machine Learning to recommend products to their customers. The basic idea of this kind of recommendation comes from collaborative filtering. Collaborative filtering is the process of comparing users with similar shopping behaviors in order to recommend products to a new user with similar shopping behavior.
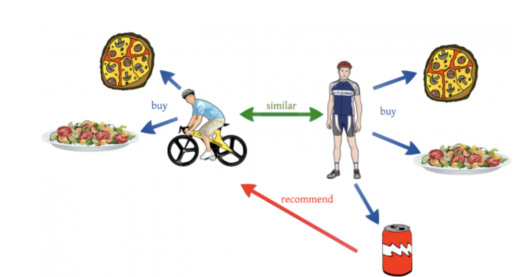
To better understand this, let’s look at an example. Let’s say a user A who is a sports enthusiast bought, pizza, pasta, and a coke. Now a couple of weeks later, another user B who rides a bicycle buys pizza and pasta. He does not buy the coke, but Amazon recommends a bottle of coke to user B since his shopping behaviors and his lifestyle is quite similar to user A. This is how collaborative filtering works.
With this, we come to an end of this blog. I hope these Machine Learning Interview Questions will help you ace your Machine Learning Interview.
If you have any suggestions or comments.Please let me know.....
No comments:
Post a Comment